Our visual cortex is organized to provide useful information about the 3D world around us. This includes information about the locations, identities, surface textures, shape, and graspability of objects, about the location, posture, and actions being performed by people around us, and about the 3D structure of our local visual environment, and how it fits into a larger map of physical space. Our lab uses a combination of fMRI (brain scans), psychophysics (behavioral experiments), and computational modeling to study how information about objects, bodies, and scenes is represented in the brain.
Our ultimate goal is to understand how these various visual representations – these pieces of your mind – are affected by attention and used in visual cognition, including visual imagination, navigation, and reasoning about 3D objects.
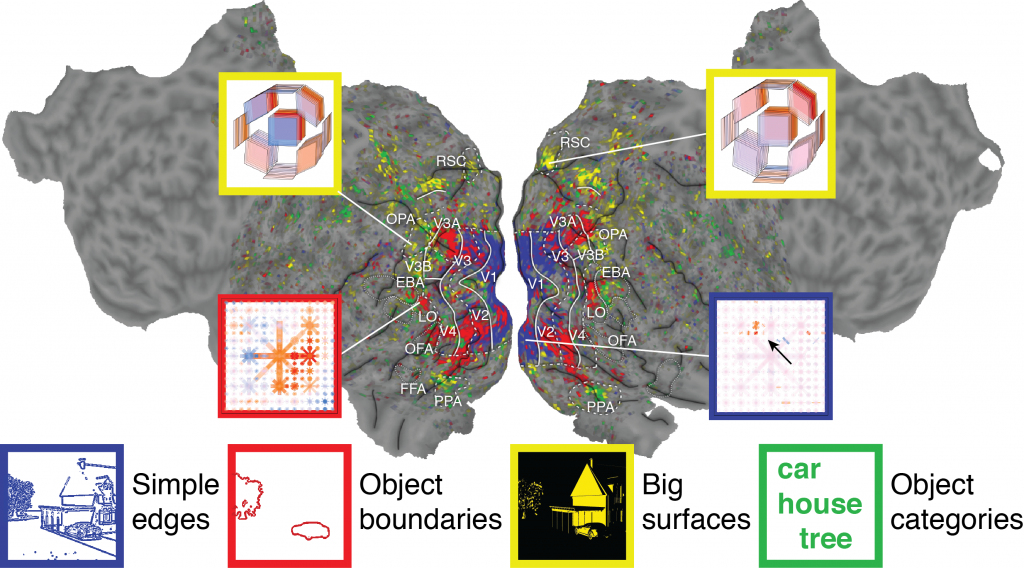
The image above is a flattened map of a brain. Each color shows parts of the brain that are sensitive to particular kinds of information, and the insets show our best guesses at the specific information contained in the tiny (3mm3) bits of the brain indicated by the pointer lines.
Scene representation
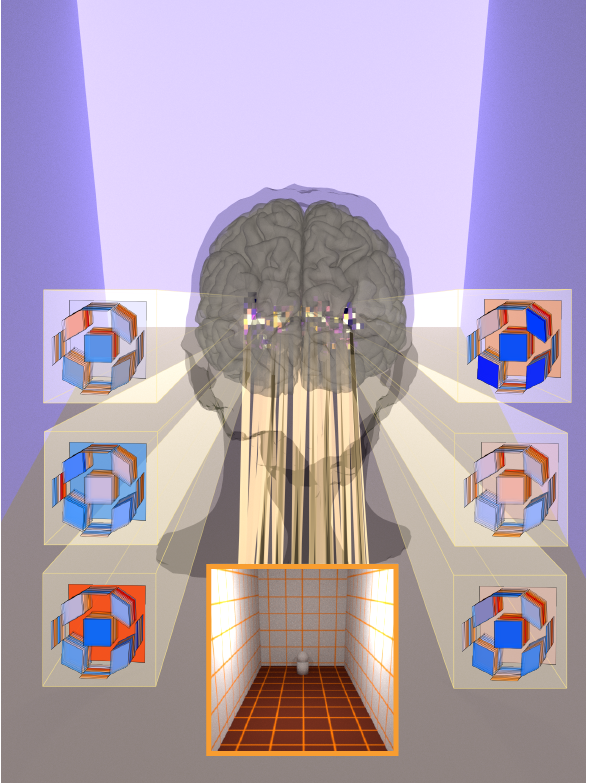
In recently published work, we explored how scene-selective areas represent the 3D structure of visual scenes. We found that individual voxels in scene-selective areas are selective for particular combinations of the orientation of and distance to large surfaces in scenes. Check out this interactive brain viewer to see how well our model performed, how individual voxels are tuned for different aspects of 3D structure, and for an overview of the work.
This work has relied on graphics software to determine the orientation of surfaces in each stimulus. In ongoing work, we plan to compute surface normals and other structural features of scenes directly from stimulus pixels using deep neural networks.
Object representation: boundaries
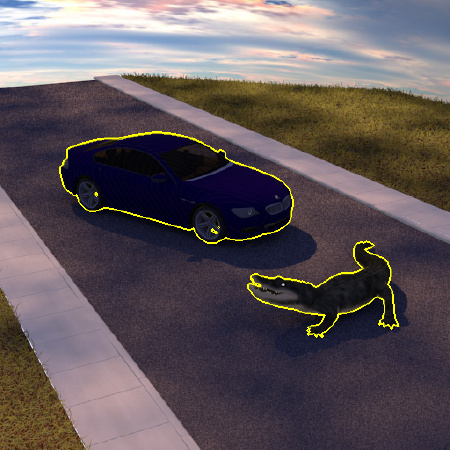
Boundaries between objects and scene backgrounds have long been hypothesized to be a critical element of object representations in the brain. In ongoing work, we have sought to determine how much brain response variance can be predicted based on object boundaries vs based on other features (such as motion, semantic features, or features extracted from deep neural networks).
Object representation: volumetric shape
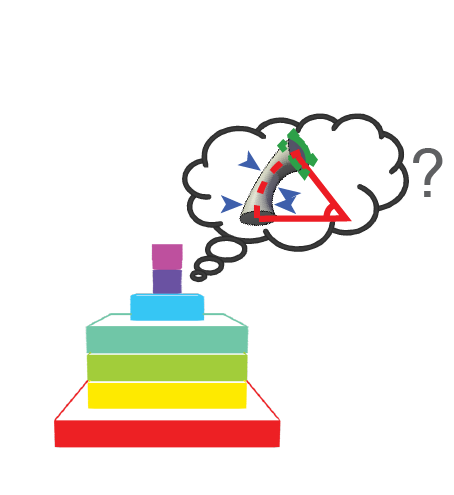
We are currently exploring how shape is represented in deep neural networks, with the ultimate aim of using representations of shape derived from deep neural networks (DNNs) to model brain activity. We have used a highly varied set of shape stimuli (geons) to test whether DNNs can achieve human-level shape recognition performance. Briefly, we find that if DNNs are trained on a sufficiently large dataset of shapes, they can perform impressively well, but they do not achieve human-level accuracy when trained on standard datasets.
Other projects
The lab is currently building capacity (in people, data, and software) to address questions relating to attention to objects and scenes, as well as to model responses to bodies and actions. If you are interested in working in these areas, the lab is currently looking for a research assistant or postdoc; potential graduate students are encouraged to apply for the UN Reno graduate program in Neuroscience or Psychology – Cogntive & Brain Sciences.
Please contact Dr. Lescroart for more information about any of these projects and/or about positions in the lab.